 |
D-Lib Magazine
July/August 2013
Volume 19, Number 7/8
Table of Contents
Using Data Curation Profiles to Design the Datastar Dataset Registry
Sarah J. Wright, Wendy A. Kozlowski, Dianne Dietrich, Huda J. Khan, and Gail S. Steinhart
Cornell University
Leslie McIntosh Washington University School of Medicine
Point of contact for this article: Sarah J. Wright, sjw256@cornell.edu
doi:10.1045/july2013-wright
Printer-friendly Version
Abstract
The development of research data services in academic libraries is a topic of concern to many. Cornell University Library's efforts in this area include the Datastar research data registry project. In order to ensure that Datastar development decisions were driven by real user needs, we interviewed researchers and created Data Curation Profiles (DCPs). Researchers supported providing public descriptions of their datasets; attitudes toward dataset citation, provenance, versioning, and domain specific standards for metadata also helped to guide development. These findings, as well as considerations for the use of this particular method for developing research data services in libraries are discussed in detail.
Introduction and Background
The opportunities afforded for new discoveries by the widespread availability of digital data, research funders' evolving policies on data management and sharing, including the US National Science Foundation and the National Institutes of Health, and the roles of academic libraries in facilitating data management and sharing have attracted a great deal of attention in the past several years.1 Libraries and librarians have embraced a variety of opportunities in this arena, from conducting research to understand data management and sharing practices of researchers, to education and outreach on funder requirements.2 Some organizations are developing web-based tools to support data management planning, such as the Data Management Plan Online tool (DMP Online) with a U.S. version supported by the California Digital Library and partners (DMPTool); others are developing research data infrastructure to support data curation and eScience, such as Johns Hopkins University's Data Conservancy, the Purdue University Research Repository, and Rutgers' RUresearch Data Portal.3
One of Cornell University Library's (CUL) efforts in this area of digital data discovery is the Datastar project.4 In its first incarnation, DataStaR was envisioned as a "data staging repository," where researchers could upload data, create minimal metadata, and share data with selected colleagues. Users could optionally create more detailed metadata according to supported standards, transfer the dataset to supported external repositories when they were ready to publish, and obtain assistance from librarians with any of these processes. The system was based on the Vitro software, a semantic web application that also underlies the VIVO application, developed at CUL's Mann Library.5 A prototype system with capabilities for specialized metadata in ecology and linguistics was successfully developed, and its use piloted as a mechanism for deposit of data sets to the Data Conservancy and Cornell's eCommons repository.6 We found, however, that the conversion of these specialized metadata schemas and standards into semantic ontologies and the development of reasonably user-friendly editing interfaces based on these ontologies to support the entry of detailed metadata were far too labor-intensive to be realistically implemented or sustainable and that phase of the project has been brought to a close.
In reconceiving Datastar, we chose to focus on the idea of developing a research data registry to support basic discovery of data sets. Prior research showed that Cornell researchers would be interested in a basic data registry to demonstrate compliance with funders' requirements, and early work at the University of Melbourne which extends VIVO to accommodate basic data set description also stimulated our thinking in this area.7 The current project will transform Datastar, extending the VIVO application and ontology to support discipline-agnostic descriptive metadata, enabling discovery of research data and linking this research data with the rich researcher profiles in VIVO. Adapting VIVO for this purpose confers the advantage of exposing metadata as linked open data, facilitating machine re-use, harvesting, and indexing. As part of this project, we will develop and document a deployable and open-source version of the software. Cornell, in partnership with Washington University at St. Louis, is continuing development of the Datastar platform with this new focus.
With this core purpose of data discovery in mind, we wanted to ensure development decisions were driven by real user needs. To that end, we used the Data Curation Toolkit to conduct interviews and create a set of Data Curation Profiles (DCPs) with participants selected at Cornell University (CU, Ithaca, NY) and Washington University in St. Louis (WUSTL, St. Louis, MO).8 Others have used the Data Curation Profile Toolkit to understand researchers' data management and sharing needs and preferences more broadly, but to the best of our knowledge, ours is the first application of the toolkit to inform software development for a particular project.9 We report our findings regarding researcher data discovery needs vis-à-vis the evolving Datastar platform and our experiences with this particular method for informing software development.
Methods
We conducted structured interviews with researchers at CU and WUSTL using the Data Curation Profile (DCP) interview instrument to inform the ongoing development of a data registry.10 We conducted our interviews according to the procedures outlined in the DCP Toolkit v1.0, with minor modifications to the interview instrument.11 Modifications were made after reviewing the interview worksheet to determine whether it included questions that would help us prioritize development decisions. We identified those that did, and inserted four additional questions. No questions were eliminated from the instrument, even though some were not directly applicable to our immediate investigation. The questions added and the corresponding interview modules were:
- Please prioritize your need for... the ability to create a basic, public description of (and provide a link to) my data. (Module 4, added to question 4)
- Please prioritize your need for... the ability to easily transfer this data to a permanent data archive. (Module 5, added to question 2)
- Please prioritize your need for... the ability to track data citations (Module 11, added to question 1)
- Please prioritize your need for... the ability to track and show user comments on this data (Module 11, added to question 1)
Creation of Data Curation Profiles
Interviews were conducted by CUL and WUSTL librarians as described in the DCP User Guide; interview subjects were identified by the librarians after Institutional Review Board approval was obtained at each institution. Participants from a broad range of disciplines and data interests were invited to participate, and eight completed interviews (Table 1). All interviews were recorded (audio only), and interviews were transcribed when the interviewer indicated information was not robustly captured from the interview worksheet or notes. Each interviewer constructed a DCP according to the template in the toolkit. Participants were given an opportunity to suggest corrections to the completed profiles, names and departments were removed from the profiles, and the resulting versions were made available both on the Data Curation Profile website and Cornell's institutional repository.12
University
|
Research Topic
|
DCP Filename
|
Cornell University
|
Biophysics
|
CornellDCP_Biophysics.pdf
|
|
Sociology / Applied Demographics
|
CornellDCP_Demographics.pdf
|
|
Ecology & Evolutionary Biology / Plant Biology
|
CornellDCP_Herbivory.pdf
|
|
Genetics / Plant Breeding
|
CornellDCP_PlantBreeding.pdf
|
|
Linguistics
|
CornellDCP_Linguistics.pdf
|
Washington University in St. Louis
|
Archaeology / GIS
|
WashUDCP_GIS.Archaeolog.pdf
|
|
Physical / Theoretical Chemistry
|
WashUDCP_TheoreticalChemistry.pdf
|
|
Public Health Communication
|
WashUDCP_PublicHealth.pdf
|
Table 1: Participant interview research topics and completed Data Curation Profile file names in Cornell's eCommons repository.
Analysis and Interpretation of Completed Data Curation Profiles
Much of the information contained in the DCPs could help shape both the current software development project and other future data curation services. Therefore, it was important to evaluate and prioritize the information gleaned from the DCPs in order to guide Datastar development.
The analysis team (librarians and additional project personnel) performed an initial analysis of the profiles upon their completion. First, the team read selected sections from the completed profiles with each section assigned to two readers. Each reader individually summarized any observed trends in the information collected, identified the most useful information that might guide development and identified the most interesting results without specific regard for Datastar. Each pair of readers then jointly reached agreement on these topics and shared the findings with the analysis and development team, which produced a summary of findings for internal use.
Second, we evaluated the quantitative responses from the interview worksheets. All questions with discrete responses (e.g. prioritization of service needs; yes/no) were evaluated using an assigned numerical value system. For the prioritization questions, "Not a priority" was assigned a value of 0, "Low Priority" a 1, "Medium Priority" a 2, and "High priority" a 3. For the yes/no style questions, a "no" was assigned a value of 0 and a "yes" a 1. Responses of "I don't know or N/A (DKNA)" and instances where no response was recorded were also tabulated; questions where such responses were greater than 25% (i.e. greater than two of eight profiles) of the total were discussed. These numbers were used to calculate the average prioritization value (APV), which represents the overall desire for a given service and helped guide further discussion during analysis.
This value system was not meant to show statistical differences but was used to evaluate the relative importance of these services to the researchers and reflect an overall prioritization of the entire pool of respondents. After determining the average prioritization values, we reviewed the verbal interview answers to confirm the replies.
Relevant Findings and Discussion
Key findings regarding data sharing and the relationship of scientific research data to institutional repositories are covered in Cragin et al.13 Our findings with respect to data sharing reinforce many of the general conclusions in that paper and will not be detailed here. Instead, our focus is on those issues that directly influenced the current iteration of Datastar or will be considered for future development of Datastar.
Findings That Directly Influenced Current Datastar Development
After evaluation and prioritization of the findings from the interviews, a set of responses that were particularly relevant to the current iteration of Datastar emerged. These findings are summarized in Figure 1.
Figure 1: Summary of prioritization responses for features included in the current version of the Datastar registry. Asterisk (*) denotes a question added to the original DCP Tool for this project. Total number of interviewees in all cases was eight.
Discovery and a Basic Public Description
One of the primary goals of Datastar as a data registry is to have it serve as a hub for discovery of that research data. Some of the more traditional ways of locating data of interest to researchers have included direct contact with peers or collaborators or finding related work via publications. However, the large and quickly growing volume of information available about scientific efforts and accomplishments now available on the Internet has opened a new route of discovery: Internet search engines. One of the simplest elements that can be used to facilitate Internet discovery is a basic description of the item (in this case the dataset) of interest.
To learn how to make Datastar effectively support data set discovery, we asked researchers about the importance of Internet discoverability, types of metadata elements to enhance discovery, and more generally, how they envisioned prospective users finding their data sets.
First, researchers were asked to prioritize "the ability for people to easily discover this dataset using Internet search engines (e.g. Google)." The response was strongly in favor of Internet discoverability: six respondents rated it as high or medium, one ranked it of low importance and one researcher did not reply. Other routes of discovery that were mentioned were individual researcher web pages, laboratory web pages, department web pages, Google Scholar, Web of Science, and domain-related web pages (e.g. "language page" for the linguist, web interfaces for GIS data for the archaeologist). For the researcher who ranked Internet discoverability as low, it is important to note that the researcher felt "confident that the existing channels of data distribution (both online and print) were sufficient to ensure the farmers, breeders and researchers in counterpart programs in other agricultural extension services were discovering the data"; in this case, the need for broader discovery was perhaps not prioritized because the current system was deemed adequate.14
Second, researchers were asked the open-ended question, "How do you imagine that people would find your data set?" The demographer was already aware that the project website was found online by searching for the project name or for terms related to the project topic such as "county population" or "projection".15 The ecologist studying herbivory mentioned that a basic public description could aid in discoverability, drawing a parallel to literature database searches that are done on geographic location or period of time in conjunction with a basic project description (i.e. topic). This researcher specifically mentioned the idea of using a "sort" or "find more data like this" tool or using descriptive terms such as keywords, species and geographical inputs.16 While a primary set of attributes useful for description and discovery had already been identified (e.g. author, title, subject), the interviews confirmed those attributes and identified the additional facets geography and time, which were incorporated into the current version of Datastar. Future iterations of Datastar may also include search terms like species or other facets that would enable researchers to "find more data like this."
Finally, researchers were asked to prioritize "the ability to create a basic, public description of (and provide a link to) my data." The positive response six researchers ranked it high or medium priority, one answered it was not important, and one researcher did not answer the question indicated that a publicly accessible data registry would likely be of interest to researchers.
When asked "Who would you imagine would be interested in this data?" the majority of respondents (five) felt their data could potentially be used by groups requiring general public access such as "students," "farmers," "policy overseers," "language learners," and "educators." More than half of researchers interviewed believed their work to be applicable to such broad-range audiences; this reinforces the need for a means of easy, public discovery of current work and data in a wide variety of fields. The responses to all these questions are consistent with the current development trajectory for Datastar.
Data Citation and Citation Tracking
Scholarly manuscript citations have quickly become "the currency of science", with research resources dedicated to tracking, monitoring and linking of publications (e.g. Scopus, Web of Science, Google Scholar, Journal Impact Factors).17 More recently, citation of datasets has gained attention of this kind as well. Web of Knowledge has added a new database, the Data Citation Index, which is designed to help users discover datasets and provide proper attribution for those datasets.18 DataCite, established in 2009 and with member institutions around the world, is another effort to support data archiving and citation.19 Proper, consistent, relevant citation of a dataset serves multiple purposes. From the researcher perspective, dataset citation can allow proper credit to be given to both the original researcher and, when applicable, to the dataset source. A critical step in the process of scientific collaboration and data sharing, dataset citation forms a connection between the data and related publications, as well as a connection between related datasets. From a data management perspective, citation lays the foundation and framework for capturing relationships between datasets, publications and institutions, enabling further analysis of the impact and usage of the data.
When asked to prioritize "the ability to enable version control for this dataset," five researchers considered this to be a high priority and one, who ranked version
control as not important, felt the "data do not have different versions". The ecologist felt strongly that "the link between the data and the related publication(s) is very important" and went so far to say that he "would like any repository to provide the link and to make that linking easy".20 Similarly, the biophysicist indicated that "the ability to cite the publicly available protein crystal structure is a high priority, as it will be referenced in the published paper and others should be able to find it".21
While the ability to easily create a citation for a dataset was prioritized highly, on average the need to track citations was ranked as not quite as high an importance (APV for tracking citations was 2.29 compared to 2.43 for ability to cite the data). Five of the eight researchers interviewed ranked tracking citations of medium importance, two ranked it of high importance and one researcher did not know how important it was to him personally, "since he believed people would do this regardless". For those who did rank it as a high priority, one researcher did specifically mention that "he considers this the real measure of the value of his data".22
Prior to the interviews, providing a means for citing described datasets was being considered for inclusion in Datastar. The researchers made clear its importance and a recommended citation format for the dataset(s) will be a future feature in the application.
Versioning
A natural part of the lifecycle of many datasets is the potential for re-processing, re-calibration or updating, either of a part or of the whole file. For example, remote sensing data from satellites is often released near real-time; it is not uncommon for instrument calibration changes to be applied to those data after the initial release, requiring the dataset, or a portion thereof, to be re-processed to reflect the correct instrument parameters. In other datasets, errors can be found and corrected, or versions can be created for restricted use access that are different than those destined for public access; data can also be appended or added, keeping a time-series up to date or supplementing information already within the dataset. Tracking of such changes can be important when trying to re-create an analysis or interpret an outcome, and ensures long-term usability of a dataset.
When asked to prioritize "the ability to enable version control for this dataset," five researchers considered this to be a high priority and the one, who ranked version control as not important, felt the "data do not have different versions".23 Interestingly, the same researcher mentioned "new data is being added to the data set but no data are being changed"; it is possible that the researcher had a different concept of versioning that did not include time series data. Both the plant geneticist and the biophysicist mentioned their labs presently employ protocols that track data versions; for those researchers and others like them who already maintain records of changes and modifications, transfer of this information to a data registry should be a logical next step. Interestingly, while the biophysics lab did track data processing versions, the project Principle Investigator felt this was more critical to internal use of the data, rather than of the shared version. Nonetheless, Datastar development plans include offering the ability to record information about dataset version or stage.
Provenance
Key to the success of sharing and re-using data is the user's confidence in the quality of the datasets; with the advent of publicly available and openly shared data, the risk of not knowing the source of and possible changes to a dataset before use is greatly increased. This process of documenting the origins of and tracking movements, transformations and processes applied to a dataset is called data provenance.24
To determine whether data provenance is important to researchers, we asked them to prioritize their need for "documentation of any and all changes that were made to the dataset over time." This documentation of changes or provenance of the dataset was ranked as a high priority by three researchers interviewed, with one specifically mentioning the desire to know "whether any changes had been made, and by whom".25 In the case of the ecologist, data provenance was only a medium priority, but this was "because he didn't feel like any changes should be made" to the dataset.26 The biophysicist stated data provenance was not applicable to his dataset, because "all processing and re-processing of the data starts from the initial files... and progresses linearly through the data stages". In this case, the researcher did feel that preservation of such initial files was "critical for internal use" but not necessarily of importance as "part of the scientific record".27
Given the combination of the range of responses to the idea of tracking provenance with the technical demand of a full implementation of workflow provenance, Datastar will have the ability to record whether or not a dataset is the original version or the derived version and to document the originating dataset for a derived version.28 Datastar will not automatically capture this information; responsibility for recording provenance will rest in the hands of those who submit the data.
Formal Metadata Standards
The application of formal metadata standards to datasets establishes the framework on which both the technical and practical implementation of organization and discovery can be built.29 Numerous metadata standards applicable to scientific data exist (e.g. Darwin Core, Federal Geographic Data Committee Content Standard for Digital Geospatial Metadata (FGDC/CSDGM), Ecological Markup Language, Discovery Interchange Format, ISO 19115, etc.), but when specifically asked to prioritize their need to "apply standardized metadata from your field or discipline to the dataset," only one researcher felt this was a high priority, three said it was a low priority, and the remaining half of the researchers either did not know or did not respond. Looking at the pool of DCP's that have been completed at other institutions, similar responses are common, with seven of thirteen profiles not mentioning the prioritization of standardized metadata application.30
This notable lack of response or interest in formalized metadata standards could be due to a variety of reasons. It is possible that no domain-specific metadata standards exist in the participants' respective fields or that they fail to use standards that do exist. Failure to use existing standards could simply be due to a lack of awareness, or because the importance and function of metadata may not be valued by busy researchers. There are barriers to the use of metadata standards by non-experts some standards exist but lack openly available and usable tools (e.g. Data Documentation Initiative (DDI)), or are complex and difficult to use (e.g. DDI, FGDC/CSDGM).
Another likely reason for the researcher responses could be one of semantics. The word "metadata" may not be understood across subject domains; sometimes "documentation" or "ancillary data" or another domain-specific term is more readily recognized by researchers when talking about scientific metadata. Indeed, while some locally developed standards, such as meta-tables, geo-referencing and data diaries are regularly employed, no researcher in this study mentioned any formal metadata standards that were currently being used on their datasets.31 The linguist did identify this lack of metadata standardization as a problem, adding that it "may be partially due to the very narrow and specific nature of each researcher's project".32 Similarly, another researcher said that no formal standards were applied to describe or organize their data because of "the highly specialized and unique nature of the lab's research".33
Although responses were few and mixed, we feel that there is substantial doubt surrounding the reasons for this lack of interest in standardized metadata. Therefore, Datastar will provide the option to provide information about formal metadata standards used to describe the datasets. If the researcher chooses to use a metadata standard, that information can be leveraged and becomes an attribute of the dataset itself. We believe that this additional, optional descriptive facet will enhance data discovery, one of the basic functionalities of the Datastar registry.
Findings That May Influence Future Datastar Development
Some findings were beyond development capabilities for the current iteration of Datastar. They were still useful because they helped to provide additional context and details about the ways researchers prefer to interact with their data. Many of these findings were related to tools used to generate and use data, as well as linking and interoperability of data. Although not all of these findings will result in new functions in later versions of Datastar, the answers to these questions will almost certainly direct future Datastar development. These findings are summarized in Figure 2.
Figure 2: Summary of prioritization responses for features not necessarily implemented in the Datastar registry. Asterisk (*) denotes a question added to the original DCP Tool for this project. Total number of interviewees in all cases was eight.
Connecting or Merging Data
The overarching goal of Datastar is to improve discoverability, and in turn, re-use of data sets. A primary way that data sets are re-used is by connecting and merging them with other related data sets, whether that relationship is topical, geographic, temporal or through some other affiliation.
Among the different services associated with tools, linking and interoperability of datasets, the "ability to connect or merge your data with other datasets" emerged as the highest priority. Most of the researchers (six) considered connecting or merging data a high to medium priority. Two said they didn't know or it wasn't applicable; none of the researchers interviewed considered the ability to connect or merge data either low priority or not a priority. The motivations researchers gave for merging datasets varied, although meta-analyses were cited more than once. The ecologist considered merging datasets a high priority because he already does this, but currently extracts the raw data needed to compile meta-analyses from the papers themselves. This method is less than ideal, so he is enthusiastic about a tool that would allow him to (1) identify related datasets via a "find more data like this" function and (2) access and combine the related data sets into meta-analyses.34 The sociologist's research involves acquiring demographic data from a variety of sources, processing, analyzing and aggregating the data in their own database, so merging datasets is a very high priority for this research group as well.35 In contrast, one of the few researchers interviewed who did not identify merging or connecting datasets as a priority, explained that this was due largely to the specificity of the research project and a lack of standardization in the field.36
It is worth speculating whether Datastar can provide services in this arena beyond the basic service of fostering discovery. As a registry, Datastar is not focused on storing the data itself and will not support data integration, analysis, or comparison tools in this phase of development. Using Datastar, one will be able to find information about the dataset, including where it is located, but will not be able to manipulate the datasets themselves. By improving discoverability, Datastar will have the potential to aid with activities such as meta-analyses by improving researchers' abilities to find related datasets.
Data Visualization and Analysis
There are many commercial and open source tools used to analyze and visualize data (e.g. Open Refine, R, ArcGIS, and many more). Half the participants reported using Excel, both to analyze and to create charts and graphs. A variety of other tools were mentioned by researchers, including Google APIs, SAS, Matlab, instrument-specific software and proprietary code generated by the lab.
It was not surprising then, that when asked to prioritize their need for "the ability to connect the data set to visualization or analytical tools" it emerged as a high priority. Four researchers considered this a high priority and three a medium priority; only one, the public health communications researcher, considered it a low priority. Interestingly, the same researcher uses a number of sophisticated software programs for analyses and data visualization. The demographer, who considered data visualization and analytical tools a high priority, reported "if the data were hosted in an external repository, it would be high priority to be able to continue to use visualization tools such as Google Maps and Google Charts".37
Because the need for analysis and visualization of data was highly ranked by the majority of the researchers interviewed, we are exploring the types of support Datastar, as a dataset registry, might be able to provide. One consideration may be to employ visualizations for the discovery of datasets such as interactive maps or timelines showing the relationships between registered datasets. More exploration and thought will be required before we attempt to address these needs.
Usage Statistics
Website useage statistics are commonplace. In the library environment, usage statistics are frequently used for collection development decisions and recent investigations have focused on exploring whether a new measurement of journal impact might be based on electronic usage statistics instead of the typical measure of citation frequency.44 Researchers, however, largely rely on citations as their measure of success, so it was of interest to learn whether they prioritized the collection of usage statistics for their data.
The "ability to see usage statistics (i.e. how many people have accessed the data)" received the full range of prioritization, from not a priority to high priority. Half of the researchers (four of eight) considered collecting statistics on use a medium priority, two considered it not a priority, and one each considered it low and high priority. In contrast, the majority of the researchers interviewed considered the ability to track citations either equal to or higher priority than collecting usage statistics (APV = 2.29 vs 1.50, respectively). One researcher would like to collect both metrics in order to measure "conversion to scholarship," or how many uses of the data resulted in publications or other tangible scholarly products.45 The demographer already tracked usage of the data, using Google Analytics on the project website; this researcher's highest priority is tracking "the internet domains of users and the referring keywords used to find the website".46
These comments are indicative of a general attitude that citations are the basis of the scholarly record, while usage statistics are seen as a less important, administrative function. It was interesting to note that every researcher answered the question, indicating that they were familiar with the concept of collecting usage statistics. One researcher even said it and citation tracking were of low importance, because "people would do this regardless".47 Given this expectation, it is probably important that Datastar give researchers some measure of data use, even if it is as basic as a report of the number of page views. This is a feature that hadn't been considered before performing the interviews, but that will be considered for future iterations of Datastar.
User Comments
User comments are not unknown in the scholarly publishing and scientific communities, but they are still a controversial idea among many researchers. It is important to consider the purpose since researchers may have very different opinions of comments in the context of a social network vs. the context of public peer review. A recent study of public peer review found that short comments submitted by the "interested members of the scientific community" were far less useful for selection and improvement than were the comments of invited reviewers.38 Furthermore, when Nature tried open peer review, they found that it was far from popular; both authors and reviewers disliked commenting on the scholarly record in this manner.39 Less formal examples of user comments can be found employed in scientific social networking sites like Nature Network, Biomed Experts and Mendeley. The purpose of the interactions in these and other social sites is generally to collaborate, post articles and trade ideas.40 Since Datastar is a dataset registry, not quite fitting in the category of peer-reviewed content or social networking site, it is an open question whether user comments would be of interest to researchers.
The interview instrument contained two questions related to user comments, one about "the ability of others to comment on or annotate the dataset" and one about "the ability to track and show user comments on [the] data." Only three researchers gave a high or medium priority to the possibility of users commenting on or annotating datasets, while four stated tracking or showing comments was at least a medium priority. Only one researcher was consistently enthusiastic about this idea and talked about the potential of user comments to provide "new life to the data, and could serve as a global virtual lab meeting".41 Two others were moderately enthusiastic, categorizing commenting or annotating a medium priority and tracking comments a high and medium priority, respectively.42 Most of the other researchers were either hesitant or completely dismissive of any possible utility of user comments; five responded that enabling user comments was either low priority or not a priority at all. One expressed concerns about enabling unmediated public comments, noting that misinformed comments can permanently tarnish a publication or dataset.43 Therefore, if user comments are a feature of a future version of Datastar, adding the ability to moderate comments should be considered. Because the researchers interviewed had very mixed opinions of these services, user comments are a possible future development, but are not a high priority for the first iteration of the Datastar data registry.
Methodological Considerations
The Data Curation Profile Toolkit has been used to discover and explore researchers data management and sharing needs and attitudes, but to the best of our knowledge this is the first time the toolkit has been used to inform the development of a specific tool.48 We found it a useful method for informing software development because it helped to better define the research context in which the tool will potentially be used. We do have some concerns about our use of this tool. One issue already discussed to some extent in the section on formal metadata standards is that of the terminology used in the toolkit. Half of the researchers either did not answer or responded that they did not know whether they considered the ability to apply standardized metadata from their discipline a priority. This may indicate that there is not an accepted (or at least highly used) metadata standard for their area of research, but descriptive metadata also has many different names and it might have been helpful to familiarize ourselves with the research area's preferred term ahead of time (i.e. "code book", "ancillary data" or "documentation") to minimize the use of unfamiliar terminology. Indeed, several researchers discussed locally developed standards, including geo-referencing and data diaries, but only one prioritized "formal metadata standards" as it was presented in the interview questionnaire.49
Another issue was how much of an effect the interviewee's focus on one particular dataset had on the nature of the answers given. The interviews may reflect the researcher's needs and attitudes concerning one particular dataset, not the body of their research or views on data sharing in general. However, we feel that the potential pitfalls of asking about one data set are outweighed by the benefit of getting concrete answers when the researchers have a real example in mind. Also worth considering is whether responses would have differed if we had described the goal of our project, i.e. developing a dataset registry. We did not describe the current project to avoid introducing bias, however, a fuller description of the tool in development might have elicited different responses. Despite any lingering reservations, the process of interviewing researchers and creating profiles provided a useful view into a cross-section of research disciplines and helped us to gain insight into the environment in which the tool in development will be used. As libraries become increasingly involved in developing data management services and tools, methods such as this one are important to ensure that services are based on real user needs.
Conclusions
At the outset of this project, it was our goal to develop a research data registry to support basic discovery of datasets, and we had already identified interest from Cornell researchers in a data registry to demonstrate compliance with funders' requirements.50 In order to ensure development decisions were driven by user needs, we performed structured interviews using the Data Curation Profile Toolkit.51 The eight researchers interviewed represented a wide range of research areas and had a similarly wide range of priorities with respect to managing their data. Although no priorities were shared by all researchers, the process did help to identify some services that are likely to be valued and utilized across research areas. Those cross-cutting high priority services included creating a basic public description of datasets, enabling citation of datasets in publications, support for version control and requiring the citation of datasets by users. Several of these had already been identified as priorities in the current phase of Datastar. The interviews provided affirmation that these services were indeed prioritized by researchers in different fields.
In addition to confirming ideas regarding researcher priorities, the interviews also helped identify additional services important to researchers, including data citation and discovery by web search engines, both of which can be incorporated in the current iteration of Datastar. While these researchers also prioritized the ability to connect and merge data sets and to perform visualization and analysis of data sets, a data registry cannot support these functions.
Performing user studies at the outset of our project to redirect Datastar development allowed us to discover how researcher needs converged (and diverged) across disciplines.
This in turn informed our decisions regarding how DataStar can best balance the needs and interests of those sharing their data, support requirements of funding agencies, and incorporate emerging organizational principles of linked open data, facilitating machine-reuse, harvesting, and indexing. As one might have expected, we were also left with more questions and more ideas for future projects and tool development to foster data stewardship.
Acknowledgements
We thank Kathy Chiang, Keith Jenkins, Kornelia Tancheva, and Cynthia Hudson for completing Data Curation Profiles for this project, and are grateful for additional assistance from Jennifer Moore, Robert McFarland and Sylvia Toombs.
References
-
National Science Foundation, "Dissemination and Sharing of Research Results"; National Institutes of Health, "NIH Data Sharing Policy".
-
Catherine Soehner, Catherine Steeves, and Jennifer Ward, E-Science and Data Support Services: A Study of ARL Member Institutions, Washington, DC: Association of Research Libraries, 2010.
-
Martin Donnelly, Sarah Jones, and John W. Pattenden-Fail, "DMP Online: The Digital Curation Centre's Web-Based Tool for Creating, Maintaining and Exporting Data Management Plans", International Journal of Digital Curation 5, no. 1 (2010); California Digital Library, "DMPtool: Guidance and Resources for Your Data Management Plan"; Johns Hopkins University, "Data Conservancy"; Purdue University Libraries, "Purr: Purdue University Research Repository"; Rutgers University Libraries, "RUresearch Data Portal".
-
Dianne Dietrich, "Metadata Management in a Data Staging Repository", Journal of Library Metadata 10, no. 2 (2010): 79-98 http://doi.org/10.1080/19386389.2010.506376; Huda Khan, Brian Caruso, Jon Corson-Rikert, Dianne Dietrich, Brian Lowe, and Gail Steinhart, "Datastar: Using the Semantic Web Approach for Data Curation", The International Journal of Digital Curation 6, no. 2 (2011): 209-21 http://doi.org/10.2218/ijdc.v6i2.197; Gail Steinhart, "Datastar: A Data Staging Repository to Support the Sharing and Publication of Research Data", Paper presented at the 31st Annual International Association of Technical University Libraries Conference, West Lafayette, IN, June 20-24, 2010.
-
Cornell University Library, "Vitro: Integrated Ontology Editor and Semantic Web Application"; Medha Devare, "VIVO: Connecting People, Creating a Virtual Life Sciences Community". D-Lib Magazine 13, no. 7/8 (2007). http://doi.org/10.1045/july2007-devare
-
Khan, Caruso, Corson-Rikert, Dietrich, Lowe, and Steinhart, "Datastar: Using the Semantic Web Approach for Data Curation", 209-21.
-
Gail Steinhart, Eric Chen, Florio Arguillas, Dianne Dietrich, and Stefan Kramer, "Prepared to Plan? A Snapshot of Researcher Readiness to Address Data Management Planning Requirements", Journal of eScience Librarianship 1, no. 2 (2012) http://doi.org/10.7191/jeslib.2012.1008; University of Melbourne, "VIVO & ANDS: Building a Turnkey Research Data Metadata Store on VIVO".
-
Michael Witt, Jacob Carlson, D. Scott Brandt, and Melissa H. Cragin, "Constructing Data Curation Profiles", International Journal of Digital Curation 4, no. 3 (2009): 93-103. http://doi.org/10.2218/ijdc.v4i3.117
-
Purdue University Libraries, "Data Curation Profiles Toolkit"; Melissa Cragin, Carole L. Palmer, Jacob R. Carlson, and Michael Witt, "Data Sharing, Small Science and Institutional Repositories", Philosophical Transactions of the Royal Society A: Mathematical Physical and Engineering Sciences 368, no. 1926 (2010): 4023-38; Witt, Carlson, Brandt, and Cragin, "Constructing Data Curation Profiles", 93-103.
-
Ibid.
-
Purdue University Libraries, "Data Curation Profiles Toolkit".
-
Kathy Chiang, Dianne Dietrich, Keith Jenkins, Kornelia Tancheva, Sarah Wright, Leslie McIntosh, Cynthia Hudson, and J. Moore, "Data Curation Profiles Completed for the Datastar Project", eCommons@Cornell University Library, http://hdl.handle.net/1813/29064.
-
Cragin, Palmer, Carlson, and Witt, "Data Sharing, Small Science and Institutional Repositories", 4023-38.
-
Chiang, Dietrich, Jenkins, Tancheva, Wright, McIntosh, Hudson, and Moore, "Data Curation Profiles Completed for the Datastar Project", Plant Breeding.
-
Ibid. Demographics.
-
Ibid. Herbivory.
-
Eugene Garfield, "The Use of Journal Impact Factors and Citation Analysis for Evaluation of Science", Presented at Cell Separation, Hematology and Journal Citation Analysis, Rikshospitalet, Oslo, 1998.
-
Thompson Reuters, "Thomson Reuters Unveils Data Citation Index for Discovering Global Data Sets".
-
DataCite, "DataCite: Helping You to Find, Access, and Reuse Data".
-
Chiang, Dietrich, Jenkins, Tancheva, Wright, McIntosh, Hudson, and Moore, "Data Curation Profiles Completed for the Datastar Project". Hebivory.
-
Ibid. Biophysics.
-
Ibid. Herbivory
-
Ibid. Linguistics.
-
Peter Buneman, S. Khanna, and W. C. Tan, "Why and Where: A Characterization of Data Provenance". Paper presented at the 8th International Conference on Database Theory (ICDT 2001), London, England, 2001; Peter Buneman, and Susan B. Davidson, "Data Provenance - the Foundation of Data Quality".
-
Chiang, Dietrich, Jenkins, Tancheva, Wright, McIntosh, Hudson, and Moore, "Data Curation Profiles Completed for the Datastar Project". Demographics.
-
Ibid. Herbivory.
-
Ibid. Biophysics.
-
Shirley Cohen, Sarah Cohen-Boulakia, and Susan B. Davidson, "Towards a Model of Provenance and User Views in Scientific Workflows", Presented at the Third International Workshop in Data Integration in the Life Sciences, Proceedings, 2006.
-
National Science Board, Dissemination and Sharing of Research Results, Washington D.C., 2011, Report No. NSB-11-79.
-
Purdue University Libraries, "Data Curation Profiles Directory".
-
Chiang, Dietrich, Jenkins, Tancheva, Wright, McIntosh, Hudson, and Moore, "Data Curation Profiles Completed for the Datastar Project".
-
Ibid. Linguistics.
-
Ibid. Biophysics.
-
Ibid. Herbivory.
-
Ibid. Demogrpahics.
-
Ibid. Linguistics.
-
Ibid. Demographics.
-
Lutz Bornmann, Hanna Herich, Hanna Joos, and Hans-Dieter Daniel, "In Public Peer Review of Submitted Manuscripts, How Do Reviewer Comments Differ from Comments Written by Interested Members of the Scientific Community? A Content Analysis of Comments Written for Atmospheric Chemistry and Physics", Scientometrics 93, no. 3 (2012): 915-29.
-
"Overview: Nature's Trial of Open Peer Review", Nature International Weekly Journal of Science (2006). http://doi.org/10.1038/nature05535
-
Lisa R. Johnston, "Nature Network", Sci-Tech News 62, no. 2 (2008): 34-35.
-
Chiang, Dietrich, Jenkins, Tancheva, Wright, McIntosh, Hudson, and Moore, "Data Curation Profiles Completed for the Datastar Project". Herbivory.
-
Ibid. Demography and Linguistics.
-
Ibid. Biophysics.
-
Oliver Pesch, "Usage Factor for Journals: A New Measure for Scholarly Impact", The Serials Librarian 63, no. 3/4 (2012): 261.
-
Chiang, Dietrich, Jenkins, Tancheva, Wright, McIntosh, Hudson, and Moore, "Data Curation Profiles Completed for the Datastar Project". Public Health.
-
Ibid. Demographics.
-
Ibid. Theoretical Chemistry.
-
Purdue University Libraries, "Data Curation Profiles Toolkit"; Cragin, Palmer, Carlson, and Witt, "Data Sharing, Small Science and Institutional Repositories", 4023-4038; Witt, Carlson, Brandt, and Cragin, "Constructing Data Curation Profiles", 93-103.
-
Chiang, Dietrich, Jenkins, Tancheva, Wright, McIntosh, Hudson, and Moore, "Data Curation Profiles Completed for the Datastar Project".
-
Steinhart, Chen, Arguillas, Dietrich, and Kramer, "Prepared to Plan?".
-
Purdue University Libraries, "Data Curation Profiles Toolkit".
About the Authors
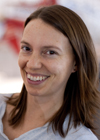 |
Sarah J. Wright is Life Sciences Librarian at Albert R. Mann Library. Her interests include data curation and scholarly communication and information trends in the molecular and life sciences disciplines. In addition to providing reference services and serving as liaison for the life sciences community at Cornell, she also participates in Cornell University Library's research support service initiatives in the area of data curation. She is a member of Cornell University's Research Data Management Services Group and Cornell University Library's Data Executive Group.
|
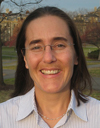 |
Wendy A. Kozlowski is the Science Data and Metadata Librarian at the John M. Olin Library, where her work focuses on issues related to the data curation lifecycle, and outreach and consultation on information management and other research data related needs. She coordinates Cornell University's Research Data Management Service Group, a campus-wide organization that links faculty, staff and students with data management services, and co-chairs the library Data Executive group. Before coming to Cornell, she spent 19 years in biology and oceanography research.
|
|
Dianne Dietrich is Physics & Astronomy Librarian at the Edna McConnell Clark Physical Sciences Library. She has scholarly communication, collection development, instruction, and reference responsibilities, and is responsible for assessing and meeting emerging data curation needs for Cornell's Physics and Astronomy communities.
|
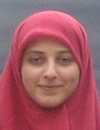 |
Huda J. Khan is the lead Datastar developer at Cornell University Library and has also worked as a developer on the VIVO project. Her work with both VIVO and Datastar delves into semantic web representations and her research background and interests include human computer interaction. Ms. Khan received her joint Ph.D. in Computer Science and Cognitive Science from the University of Colorado at Boulder.
|
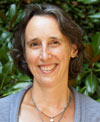 |
Gail S. Steinhart is Research Data and Environmental Sciences librarian and a fellow in Digital Scholarship & Preservation Services, Cornell University Library. Her interests are in research data curation and cyberscholarship. She is responsible for developing and supporting new services for collecting and archiving research data, and serves as a library liaison for environmental science activities at Cornell. She is a member of Cornell University Library's Data Executive Group and Cornell University's Research Data Management Service Group, which seek to advance Cornell's capabilities in the areas of data curation and data-driven research.
|
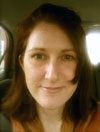 |
Leslie McIntosh oversees the medical informatics services within the Center for Biomedical Informatics, facilitating the implementation and adoption of a clinical database repository, and an in-house developed software application allowing access to the aggregated patient electronic medical records from Washington University School of Medicine and the BJC hospital system. She holds a Masters in Public Health with an emphasis in biostatistics and epidemiology in addition to a Ph.D. in public health epidemiology. Her primary interest is in facilitating medical and health research to elucidate information and knowledge from data in order to ultimately improve health.
|
|